Articles
Ways FP&A Leaders Manage the Costs of AI in Finance
- By AFP Staff
- Published: 4/2/2024
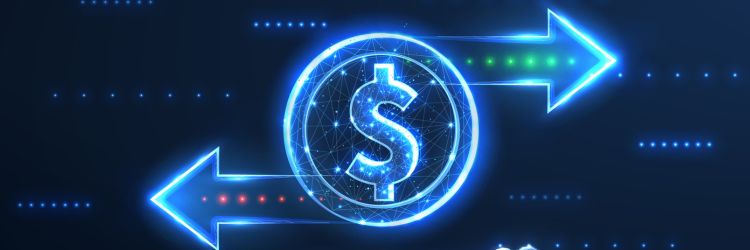
The costs associated with the use of artificial intelligence (AI) and machine learning can be numerous.
When done well, AI can give you a competitive advantage in the marketplace. But how do you manage the costs of AI to ensure the benefits outweigh the costs?
AFP’s North America FP&A Advisory Council recently met to discuss their AI goals for 2024. Included in their discussion was the potential costs of AI in finance. The list of cost considerations was rather lengthy: upfront costs, such as licensing and infrastructure; ongoing costs, such as system maintenance and model training; staffing and training costs, such as hiring experienced AI staff or upskilling current staff; data-related costs, such as data acquisition and privacy; and ethical and legal costs, such as addressing biases and putting safeguards in place to avoid mistakes or misuse.
The conversation touched on a few key themes to manage costs, such as focusing on the essentials of transparency, strategic alignment, and comprehensive planning.
One solution that is gaining in popularity and relevance is the small language model (SLM). SLMs are lightweight, generative models that encompass thousands to a few million parameters, unlike LLMs, like GPT-4, which encompass billions or even trillions of parameters. This makes SLMs cheaper to run and easier to handle.
“We're finding that you can get a lot of the same kind of results — and in some cases even better results — by training on your own smaller data sets and leveraging some of the modeling capability that we've learned in deploying large language models. It ends up being significantly faster and cheaper than using an LLM,” said one council member.
Legal fees and technical expertise are important factors when considering use cases. “For test cases, the legal and engineering considerations weighed quite heavily in our decision of which use cases would move towards the next stage,” said another council member. “Organizations are very sensitive about where they want to deploy AI capabilities at this point based on what kind of data is being used to solve a particular use case.”
Understanding your use cases and talking to your business customers about how they would leverage the opportunities you build is a critical step in managing AI costs, added another council member, who offered their own experience as a testament: “We had an idea to build a driver-based model down to the station level by employee work group type, and then forecast that out over the next 24 months for all of our operations employees. Everyone was excited. We put a team of data scientists together and probably spent half a million dollars in salary costs to build this thing out. And we ended up not using it because we couldn't explain to executives what clusters are, or random forests, or the different Python codes we use for doing the design. They just couldn't understand it and didn’t trust it.”
Another council member is seeking out ways to augment their current tools and processes with AI. “We are partnering a lot with our current vendors before seeking new ones to see how we can leverage existing tools and augment our processes within our existing ecosystem. As soon as you try to build new ecosystems, there are a lot of dependencies that stand out.”
This resonated with another council member who added, “All of the more EPM software vendors are increasingly infusing their products with AI-like capabilities, whether it's predictive analytic capabilities or the ability to ingest large amounts of data to create machine learning output.” You can wait to see what the current tide of innovation brings to you.
Another council member came up with a creative way to test the waters: They created a separate budget pool for testing AI projects, managed by a cross-functional digital decision team. The council member said, “The finance teams can experiment with [this other pot of] money without risking their own budget or P&L. It’s a more relaxed process compared to our capital governance that needs to fill out lots of forms and more process reviews. Plus, it’s a win-win: The digital decision team gets the cost savings without the project investment, and we can show others how to accelerate ideas and adoption through creative projects.”
Another important factor in managing your AI costs is transparency — and that absolutely includes your employees. “Our leadership had to be explicit in addressing the fear of layoffs and taking it out of the out of the conversation because it's real,” said one council member.
Instead, the CFO of their company refocused their rewards system in finance away from activity (the things you do) and toward impact — the value you bring to the company. “You don't get to say I spent this many hours on this thing, or I did these six things. You have to take it a step further and answer how that changed the game. How did that add value? When machine learning came along, the whole pitch was this is something that lets you focus more time on impact.”
Read More About AI in Finance
Copyright © 2024 Association for Financial Professionals, Inc.
All rights reserved.