Articles
5 Insights on AI-Powered FP&A
- By AFP Staff
- Published: 4/29/2024
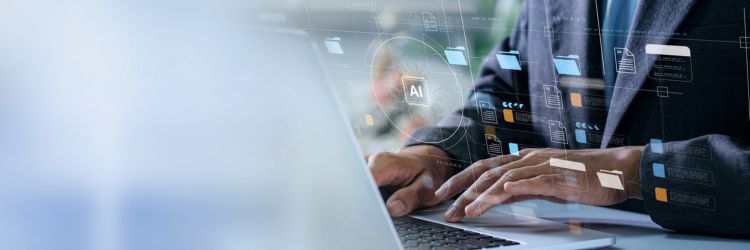
The speed at which advances in technology and automation are accelerating is unprecedented. While FP&A professionals have a strong desire to get ahead of the wave, the big question goes largely unanswered: Where do I start?
In March, AFP called in some experts to help answer this question — and many others — around AI integration with the AFP FP&A series, AI-Powered Finance: What to Do Today, exclusively sponsored by OneStream. Attendees gained an AI roadmap covering the conditions for success and learned from individual and enterprise-level case studies showcasing successful AI implementations.
Below are five key insights from the series. Recordings from the FP&A series are available to AFP members on AFP Learn. Not yet a member? Join today to get access.
Insight #1: Prepare your organization for AI with financial leadership, dedicated funding and people-driven initiatives.
In the first session of the series, “Ready, Set, AI: Embracing AI in the Finance World,” Ashok Manthena, Founder of ChatFin, and Justin Kuzma, FPAC, Senior Director of FP&A for U.S. Steel, dove into the big question around implementing AI: How to get started — and how to capitalize on steps you’ve already taken.
Manthena and Kuzma started by discussing how to successfully build an organization's foundation for an AI program. They concluded that it boils down to four primary building blocks: organization, data, expertise and the creation of policies and guidelines.
When it comes to organization, we’re speaking in the larger sense of the executive sponsorship and organizational support necessary to launch a large project. “I've seen IT teams driving the whole AI project. This structure can make it difficult to bring attention to all of the needs and challenges of finance, so it’s really helpful to have an organizational champion within finance who has an understanding of AI and its limitations,” said Manthena.
More important than the financial return on investment is the knowledge aspect, which is why U.S. Steel created separate funding and budgets for AI projects. “We dedicated some money to exploration and trying things that are new,” said Kuzma. “We didn’t want to burden managers with a potential hit to their P&L in this exploratory phase.”
U.S. Steel also upskilled its workforce to meet the demands of the new technology. “To help them see the art of the possible, we developed a program of ‘digital agents,’” said Kuzma. These are employees from across the organization who volunteer to learn about emerging technologies. “We then had them act as liaisons between our technical teams, operations teams and business groups to accelerate that adoption and push those projects forward.”
It’s important to encourage — and even push — your people to learn, grow and explore new tools and technology. Manthena said, “I always tell the finance people: ‘This is your chance! Go explore some of the use cases, build a presentation, present it to your finance leadership. Let's explore, let's see if we can bring in that efficiency, if we can close our books much faster, if we can submit that analysis much faster.’”
How should we think about our current projects to prepare for future projects with predictive and/or generative AI? Can we target doing proof of concept with a limited data set? Our speakers from the session “Ready, Set, AI: Embracing AI in the Finance World” answered these questions and more.
Insight #2: Scope the ambition of your project to set yourself up for success.
In the session “Touchless Financial Planning Through Data Science and Automation,” Josephine Schweiloch, Director of Data Science & Technology for IBM Finance, offered insights on how to drive innovation and transformation across your financial planning organization by incorporating generative AI solutions into your financial analysis and business execution.
According to Schweiloch, there are four key success factors to be considered when planning out your journey. The first is project scope.
“Start with a well-defined and actionable workflow,” said Schweiloch. “Don’t try to forecast your entire P&L right out of the gate — you're going to get stymied. It's got to be big enough to matter to your CFO or your financial analyst, so they care. But not so big that you're going to be paralyzed in pursuing it.”
To achieve this, Schweiloch advises the adoption of an MVP mindset, meaning minimum viable product. “It means don't wait to build the perfect thing; build something small and iterate. Think about your workflow orientation. Think about what your financial analysts are already doing and how you can bring technology to support it — you don't have to reinvent that. And then iterate, iterate, iterate.”
Insight #3: Generative AI is like a language calculator.
How do generative AI (GenAI) and large language models work? As Jesse Todd, Director of Finance Transformation for Microsoft, explained in the session, “Generative AI for Finance Professionals,” they’re language calculators predicting what you want to hear.
“There are three main components,” said Todd. “First, there’s the orchestrator itself, which is the brain that understands the intent of the question, figures out the context and all kinds of conceptual cues and clues, and puts them through the large language model, which is the second component. It then connects to the knowledge sources, the third component, and from there, returns the answer back to the user who can evaluate the response and modify as needed, similar to how a final reviewer would evaluate the response of a junior employee.”
According to Todd, the way in which we use generative AI falls into four groupings, which progress in complexity and value: summarization, data-driven decisioning, personalization and automation.
- Summarization: GenAI is used to summarize massive quantities of information for easier consumption and communication. One example is the summarization of earnings call transcripts to identify significant trends and highlights. Another use might be flagging important terms from customer contracts as they relate to payments, pricing and discounts.
- Data-driven decisioning: GenAI can analyze and interpret data to uncover patterns and identify trends. One way this is helpful is in analyzing variances to recommend areas of the business where variance drivers should be investigated. You could also use it to evaluate audit workpapers and resolve disputes against audit controls.
- Personalization: You can also use GenAI to tailor interactions with individualized information to create personalized output or recommendations. For example, it could be used to guide users through the process of setting up purchase orders, invoices, expenses and payments. You could also have it define a collection strategy based on a customer’s payment history.
- Automation: GenAI can streamline your operations by automating repetitive tasks, allowing your team to be more productive. One way this could play out is by automating credit checks and policy reviews to accelerate financing requests. It could also identify compliance obligations from different global jurisdictions, thereby streamlining tax and customs procedures.
How do you prevent proprietary information from making its way back to the public domain when using a large language model (LLM)? What resources are being utilized to verify the validity of the data sources used by an LLM? Jesse Todd, Director of Finance Transformation for Microsoft, explains in a Q&A on generative AI in finance.
Insight #4: AI Explainability Is Essential to Build Trust.
How do you implement an AI project when you don’t have a data scientist or AI/ML guru on staff? Kendell Churchwell, Senior Data Scientist for Southern Farm Bureau Life, and Justin Croft, Vice President of Data Science & Architecture for QueBIT, answered that question in the session “AI Projects: Four Rules for Success.”
During the session, they explained how their collaboration with external partners led to the creation of four rules for AI project success. The four rules include:
- Choose a project that matters.
- Get your data right.
- Have business buy-in.
- Explain your AI/ML.
Taking a closer look at the fourth rule, Churchwell said, “Be ready to explain and demonstrate your models. Stakeholders range from curious to skeptical, so you must be able to explain how it works. You want to have the ability to explain how the models work, why you're having to pull the data, and why you're having to make the decisions that you make.”
Churchwell explained that her team prepared executive readouts in order to educate stakeholders on predictive analytics and clarify the specifics of the business case. “You want to have some type of presentation that carries your audience through exactly what is going on in regard to the business end,” he said. “And be sure to frame model results in terms of business outcomes, not just the statistics.”
At the finish line, you want to deliver the most accurate model — a model that has the lowest error rate, depending on what you’re predicting. While that model is in the works, you will need an explanatory model, which is just like it sounds: a model that helps business owners and/or the executive team learn more about the inner workings of the model or the insights it is working to predict.
“In our case, it [the predictor] was customers,” said Churchwell. “What customers have the highest probabilities? What do they look like? What kind of clusters? What do the customers look like who are most likely to make those purchases? It may not be the model that you put in production, but it explains the inner workings.”
Insight #5: AI Will Be Built into Familiar Tools, Ready to Deploy.
There are some big questions left to be answered regarding AI, like how do we actually democratize this capability? We want it to serve the needs and capabilities of more than just the very advanced such as data scientists and ML ops teams. Historically these have been highly specialized capabilities that should be used to enable and empower the business planners.
“That’s a big element for adoption,” said Scott Leshinski, Senior Vice President of Commercial Expansion for OneStream Software, one of the speakers in the session, “AI in the Office of Finance.” “We can embed machine learning within the business planning process, and the technology and the platform that's enabling that process” rather than having to build your own AI systems.
John Behme, Director of Global Financial Systems & Analytics for Sonoco, explained that while the FP&A team doesn't need to be data scientists, they should know why specific forecasting models are used for different products. “What is driving that product's forecast? Is it an external feature? Is it an external event? Is it an internal feature, internal event?” he said.
In the course of a trial run, the team learned that federal interest rates affected the sale of strawberry containers. “It's not something we would've ever estimated or thought about,” said Behme. “It creates a really unique situation for the FP&A team to come up with creative things that they know might be driving the business, and allows us to model those and train those.”
“With better demand forecasting, we can push it straight into production,” said Behme. His team will be able to produce material based on the accuracy of these forecasts, and even purchase materials and produce product — all based on “these more accurate forecasts.”
Operations can be sped up by shortening the time between forecasting and actual production. “I think we can turn things a lot faster, and that's what FP&A wants,” said Behme. “They don’t want to be grinding out spreadsheets.”
Copyright © 2024 Association for Financial Professionals, Inc.
All rights reserved.