Articles
The Role of AI in Forecasting and Where It Falls Short
- By Vineet Jain
- Published: 9/18/2023
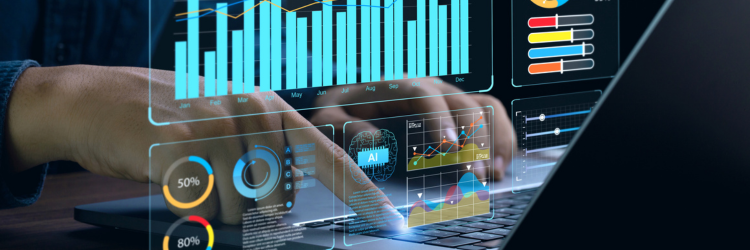
AI has the ability to process vast amounts of data at unprecedented speeds that uncover patterns and insights.
Practitioners have focused this powerful technology on reshaping how businesses approach forecasting, where the fusion of AI as cutting-edge technology with financial practices has unlocked new levels of accuracy and efficiency that make traditional methods more robust.
Historically, forecasting, budgeting and variance analysis activity heavily rely on manual efforts and reliance on historical data. With the changes in markets and complexity, the need for more agile and data-driven approaches has become paramount. AI is built with sophisticated algorithms and advanced machine learning techniques designed to process information from diverse sources that identify hidden trends and generate predictions above human capabilities.
Some of the standard methods in financial forecasting include time series, which involves observations recorded at successive time intervals; it may also leverage moving averages and exponential and rolling averages to smoothen out noise in data and identify underlying trends. AI algorithms are based on deep learning, and neural networks can identify complex patterns within such data that enable more accurate predictions of market trends, revenue, margins and other financial metrics. It constantly tests its output to self-adjust its calculations to enhance the precision of its forecasts.
Ready to learn more about AI in finance? Join us for the next AFP FP&A Series - AI-Powered Finance: What to Do Today, a complimentary virtual event exclusively sponsored by OneStream, on March 20 from 11:00 AM - 2:40 PM ET.
Examples Showcasing the Effectiveness of AI-Driven Approaches
AI comes in various forms, including robotics, machine learning, autonomous & sensor technology, computer vision and natural language processing. According to the latest data from Statista Market insights, the AI market was $108 billion in 2020 and is expected to reach $738 billion in the year 2030, which is a growth of ~683% in just 10 years.
(Artificial Intelligence - Worldwide, 2023)
These tools are becoming easier for all parts of the business to deploy; now finance has the ability to see further into the future by joining with these efforts in partnership with the business and within the office of the CFO. Here are some brief examples:
Demand Forecasting:
- E-commerce Business: An e-commerce giant heavily relies on AI for demand forecasting. Their sophisticated AI models analyze historical sales data, customer behavior, seasonality and external factors to predict future demand accurately. This allows them to optimize inventory levels, minimize stockouts and reduce excess inventory costs, resulting in improved customer satisfaction and operational efficiency.
- Healthcare: Hospitals and healthcare providers use AI to predict patient admission rates and optimize resource allocation. AI models can help hospitals adjust staffing levels, manage bed availability, etc., by considering factors like historical patient data, seasonality and disease outbreaks.
- Content Strategy: Streaming companies are utilizing AI algorithms to forecast viewer preferences and predict the success of potential content offerings. They gain insights by analyzing viewer behavior, including viewers watching habits, search history and ratings. Companies can tailor their content creation and acquisition strategies. This data-driven approach has contributed to the reputation for producing highly engaging and successful original shows and movies.
Supply Chain: Retail Inventory Management: Retail chains leverage AI to optimize inventory levels across their stores. It analyzes sales data, foot traffic, and external factors like weather. AI systems can also provide recommendations on replenishment quantities and timing. This minimizes overstocking and understocking issues that lead to improved profitability and customer satisfaction.
Operations: Energy Consumption Prediction: Utility companies employ AI to forecast energy consumption patterns. By considering historical usage, weather forecasts and economic indicators, AI models can predict peak demand periods and engage users to optimize their energy consumption. This helps prevent power shortages during high-demand periods and enhances overall grid stability.
These real-world examples underscore the transformative impact of AI-driven approaches in financial processes. By leveraging AI's analytical capability, businesses gain a competitive edge by making data-informed decisions and staying ahead of market dynamics. However, it's important to note that while AI offers tremendous potential, its success depends on high-quality data, robust model training and ongoing validation to ensure accurate and reliable results.
Common Challenges and Limitations of AI-Driven Financial Analysis
Data Quality and Quantity: In financial analysis, data quality and quantity are critical, and AI models heavily rely on data for accurate predictions. Inaccurate or incomplete data can lead to flawed outcomes, insights and predictions. Additionally, AI models require a significant amount of historical data to train effectively, and this large dataset could be a limitation for several businesses.
Model Overfitting: Overfitting occurs when an AI model performs exceptionally well on the training data but, due to exceptional transactions, fails to generalize this new, unseen data. This can happen when the model captures noise or anomalies in the training data, and the new data is widely skewed. Financial data often contains noise due to extraordinary and time-specific transactions, and without careful regularization and validation, AI models can provide misleading results.
Volatility and Uncertainty: Financial markets are inherently volatile and subject to sudden shifts due to black swan conditions, economic events or geopolitical factors. AI models might struggle to accurately predict extreme events or abrupt changes that fall outside the pattern of historical data.
Bias and Interpretability: Biases in historical data can lead to biased predictions and calculation of financial forecasts. Many AI models, particularly deep learning algorithms, operate as “black boxes,” meaning their decision-making process is complex and challenging to understand. Understanding why a model made a particular prediction is crucial for risk assessment and compliance with regulatory standards, and the biased nature impacts the confidence in the forecast.
Human Expertise and Judgment: While AI can process vast amounts of data, human expertise and judgment remain invaluable. AI may not provide the same level of analytical capability that humans have in particular situations. These financial decisions and situational nuances might be a struggle for AI models.
Regulatory and Compliance Challenges: Financial institutions are bound by regulations and compliance requirements. The regulation change occasionally makes it difficult for AI to be trained, incorporate the changes to regulations and produce correct results; therefore, this can lead to legal and reputational risks.
Cost and Implementation Complexity: AI implementation is expensive and involves high costs due to the need for data storage, computation and model deployment infrastructure. Developing, training and maintaining AI models can be resource-intensive because it requires expertise in data science, machine learning and domain knowledge, which is hard to get.
Where We Are, and Where We Are Going
While AI offers tremendous potential in financial analysis, it's essential to approach its implementation with a clear understanding of its limitations and potential risks. The above real-world examples underscore the transformative impact of AI-driven approaches in financial processes, and addressing the mentioned challenges requires rigorous data preprocessing, model validation, ongoing monitoring and expert human oversight in each area of operations.
It is interesting to refer to Gartner 2023 - Hype Cycle for Artificial Intelligence, which shows the high adoption of AI innovations and techniques and how they are going towards the peak of inflated expectations. We may be at “peak AI” hype now and we may see headlines receding. Don’t be fooled; this market is already taking off and people are hard at work finding ways to infuse AI into all parts of industry and our lives. Our role today is to acknowledge AI’s potential, work to overcome challenges, and create an AI-driven culture with a holistic adoption into day-to-day finance operations.
(Perri, 2023)
About the Author
Vineet Jain is the Director, CFO Services at Genpact, with expertise in advisory, finance transformations, and process improvements. Follow him on LinkedIn.
References
Artificial Intelligence - Worldwide. (2023, August). https://www.statista.com/outlook/tmo/artificial-intelligence/worldwide.
Perri, L. (2023, August 17). What's New in Artificial Intelligence from the 2023 Gartner Hype Cycle. https://www.gartner.com/en/articles/what-s-new-in-artificial-intelligence-from-the-2023-gartner-hype-cycle.
Rastogi, R. (2018, June 27). Machine Learning @ Amazon. The 41st International ACM SIGIR Conference on Research & Development in Information Retrieval. SIGIR '18: The 41st International ACM SIGIR conference on research and development in Information Retrieval, Ann Arbor MI USA. https://doi.org/10.1145/3209978.3210211.
Verganti, R., Vendraminelli, L., & Iansiti, M. (2020). Innovation and design in the age of artificial intelligence. The Journal of Product Innovation Management, 37 (3), 212–227. https://doi.org/10.1111/jpim.12523.
Copyright © 2024 Association for Financial Professionals, Inc.
All rights reserved.