Articles
Headcount Drivers to Manage Cash for Venture-Backed Companies
- By Renato Villanueva
- Published: 1/24/2024
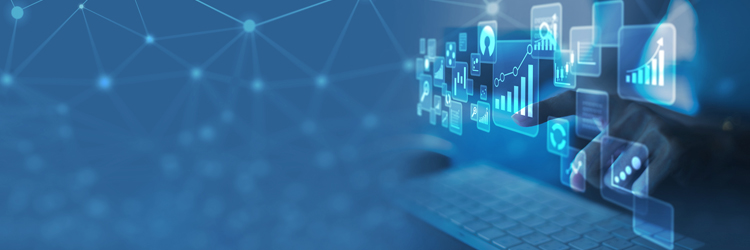
Cash is limited for startups, but with a dedicated FP&A function, companies can extend the runway, ensuring efficient operations and growth.
Managing growth is hard; FP&A helps startups design their future and connect hiring milestones.
The AFP FP&A Case Study series is designed to help you build up key FP&A capabilities and skills by sharing examples of how leading practitioners have tackled challenges in their work and the lessons learned.
This case study contains elements that are anonymized to maintain privacy and encourage open discussion.
Insight: Tying your headcount forecast to key drivers can simplify planning and create clarity across the organization.
Company Size: | Small |
Industry: | Ecommerce |
Geography: | North America |
FP&A Maturity Model: | Plan and Forecast Development |
Plan and forecast development: FP&A connects long-term strategy to current and anticipated operational activities, financial performance and risk framework; the plan is relied upon frequently in business discussions. Forecasts are honest assessments of direction, while budgets (if used) require modest effort.
Background: General Information About the Company
This case study is from my time as the Manager of Finance & Strategy at an e-commerce payment company. I joined the company when there were around 50 employees, roughly $300K in annual recurring revenue (ARR), and we were pitching for rounds of funding. Three years later, we were acquired, and the new, publicly-traded entity had more than 1,200 employees and $100 million of ARR.
When a company is in start-up mode, the minute it gets money, they have a set amount of time to get to that next milestone of customers, revenue or other benchmark in order to prove the business thesis and ability to deliver. The problem is things are going to change — constantly. And every time they do, it’s going to have an impact on how much money the company burns. Learning how to manage that burn in such a way as to get your product to market is everything.
Challenge: The Work or Difficulty FP&A Had to Address
As a company starts growing, it becomes obvious which roles need to be filled in the short term. For example, you have customers who need help solving problems that arise, so you hire customer support personnel. But how do you know how many customer support reps you’ll need over the next 2-3 years when you don’t really know your growth trajectory over that time, or even over the next 2-3 months?
As a founder myself, we are at six heads today and exploring hiring a seventh. Originally, that person was not in the plan, but the decision was made to accelerate the timeline of our product launch. But the bet we are making is whether or not giving up 5-6 months of runway to fund an additional hire will be offset by 5-6 months of product acceleration.
The role of FP&A is to find a way to gain alignment on the assumptions and add a level of predictability for the business. The model is going to be filled with a variety of assumptions, such as when you’ll start selling the product, how many customers you’ll sell to, the cost of hosting those customers, software needed to get to that point, conversion around the website and many more.
With so many unknowns, forecasting how you’ll add to the headcount over time is tricky. With every department leader asking for more, how does finance know what team should get more staff? For example, if you raised $2 million in the seed round and currently have 10 employees being paid $125,000 (fully burdened) each, you have 19 months of life. Add two employees, and that goes down to 16 months, and so on. Finance had a need to build a systematic approach to predict headcount needs that could be measured and that would provide better insight into company burn over time.
Approach: How FP&A Addressed the Challenge
We initiated a process of engaging with every business leader to understand the drivers behind their hiring needs. Different departments had different drivers for headcount requirements, for example:
- Sales is driven by quotas.
- IT has one technician for every 100 employees.
- HR has one recruiter for every 15 open requisitions.
- Customer support has one rep for every 500 accounts.
- Management has one product manager for every four engineers, and one designer for every product manager.
The insights gleaned from these interactions were utilized to develop a unified model to project headcount growth across the organization. Instead of manually maintaining a headcount forecast that requires interviews and updates throughout the year, we relied on these metrics to tell us when a new employee should be added.
The goal is to get the leader to walk away feeling like it is THEIR model. If the number of customers skyrocketed and the model said they could hire two additional people, they were approved to do so. It was their way of holding finance accountable and the way that they would feel like they were in the driver’s seat.
We recognize that not every decision can yield a clear-cut outcome, but it's crucial to engage in the process of discernment. The rationale of “we need it” is insufficient as a blanket justification. We've communicated to our leaders that we expect the unexpected and are prepared to approve budgets based on trust in certain situations. However, this approach cannot be universally applied, especially for requests involving the company's most expensive resources. A more rigorous evaluation is necessary in these cases to ensure judicious allocation of funds.
Outcome: What Came of FP&A’s Efforts and What Was Learned
As budget season rolled around, discussions centered on the accuracy and refinement of the drivers rather than the details of individual hires. For example, the Head of Customer Support explained that different-sized accounts required different amounts of attention from sales reps, so the ratio needed additional specificity that included time to resolution and type of customer. We iterated on improving the drivers and ways to track their performance.
New resource requests were analyzed in the context of their impact on headcount assumptions. For example, would Zendesk allow customer support to handle more accounts per representative? Would a transition from Hubspot to SFDC improve our sales cycle? Would an improved sales cycle lead to a decrease in SDRs? It’s all correlated.
We developed our forecasting to be less about fulfilling wish lists and more about fine-tuning the process through an increased understanding of the business. This increase in forecasting efficiency (for both finance and the business) allowed us to redirect that time to other areas, such as operational efficiency and cost control. We embraced a philosophy of ongoing advancement — learning from industry standards in order to adapt our methods to meet the changing requirements of the organization.
By focusing on cooperative, transparent forecasts, tailoring our metrics to what mattered to the business, and regularly reassessing our methods, we fostered a culture of knowledgeable decision-making that set the stage for sustainable development.
Discussion
What was your process for updating the models/ratios?
We set a rule for when to update the capacity plan. Any variance within +/- 10% wouldn’t require adjustment unless it happened more than two months in a row. We found that a single wild spike usually meant something was off in the data, and we needed to dig in to investigate. Occasionally, it would require that we adjust the headcount models.
Were there any times that the model failed, or the departments were in conflict?
The models never “failed” since the goal was to understand the business better; however, there was often a failure in communication among leadership. For example, we might add five additional salespeople to the headcount and forget to update the recruiting capacity model, or update the model and not inform everyone on the team. The times it also failed were times we failed to truly understand the dependencies.
Was there any challenge in identifying the drivers for this model?
There are some roles for which it’s simply harder to build this. For example, the role of data warehouse engineer is a highly technical role with a significant number of tasks, making it unclear what drivers are needed. We handled this manually. Overall, roughly 85% of our roles were driver-based, but there were some roles for which we would have to guess what the drivers were and enter in a few over time.
Copyright © 2024 Association for Financial Professionals, Inc.
All rights reserved.