Articles
Generative AI in Finance: 2 Big Questions, Answered
- By AFP Staff
- Published: 4/29/2024
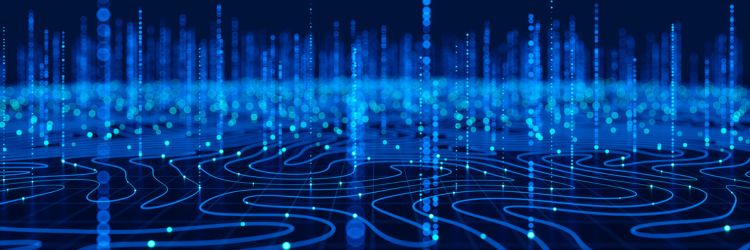
The race is on to understand how and when to apply generative AI, such as large language models (LLMs) and embedded copilots, in finance.
As part of the AFP FP&A series, AI-Powered Finance: What to Do Today, exclusively sponsored by OneStream, Jesse Todd, Director, Cross-Industry Finance Transformation, Microsoft, explained how generative AI and large language models (LLMs) work. His presentation included an overview of LLM orchestration and examples of how finance users can leverage both publicly available and private/customized LLM-assisted solutions in their work today.
Below are answers to two of the more technical questions that were asked during Todd’s session, “Generative AI for Finance Professionals.” Read more about the session in “5 Insights on AI-Powered FP&A.”
How would one prevent proprietary information from making its way back to the public domain when using an LLM?
Todd: My guidance is to leverage LLM service providers who provide access through closed APIs. This will restrict access to an LLM like a private club so only authorized users can interact with it. These APIs promise not to learn from specific user inputs, only respond to them, ensuring data privacy. Think of the data as remaining in a locked vault; the data can be viewed inside the vault by approved people (first precaution) but not removed from the vault for viewing from others (second precaution).
Examples:
- Subscription models: OpenAI’s GPT-3.5-turbo offers a subscription-based model with confidential interactions.
- Custom solutions: Some companies build their own closed APIs to safeguard proprietary data.
What resources are being utilized to verify the validity of the data sources used by LLM?
Todd: Evaluating the validity of data sources used by Large Language Models (LLMs) involves several approaches. A combination of tools and assessments ensures the reliability and trustworthiness of LLM-generated responses.
Qualitative Measures:
- Faithfulness: This refers to the accuracy of the information in the model’s responses. Researchers compare LLM-generated answers to labeled data (gold-standard answers) when available. If the model’s output aligns with these benchmarks, it demonstrates faithfulness.
- Contextual Relevance: Ensuring that LLM responses are contextually appropriate and aligned with the user’s intent. This involves assessing whether the generated text makes sense within the given context.
- Coherence: LLMs should produce coherent responses that flow logically. Incoherent or disjointed answers are considered less reliable.
Quantitative Metrics:
- Semantic Similarity: Comparing the similarity between LLM-generated responses and human-written answers. Techniques like cosine similarity or BERT embeddings help measure semantic alignment.
- Correctness: Evaluating factual accuracy. For example, if the model provides medical advice, it should be factually correct.
- Diversity: Ensuring that the model doesn’t produce repetitive or overly similar responses.
Ethical and Contextual Guidelines:
- LLMs should adhere to ethical guidelines, avoiding harmful, biased or inappropriate content.
- Context matters: Responses should consider the context of the query and avoid generating harmful or misleading information.
Availability of Labeled Data:
- When labeled data (with correct answers) is available, LLMs can be directly evaluated against these benchmarks. However, in real-world scenarios, such data may not exist.
- In the absence of labeled data, qualitative and quantitative evaluation methods become crucial.
External Toolkits and Frameworks:
- Vianai’s veryLLM Toolkit: An open-source toolkit that helps verify the accuracy and authenticity of AI-generated responses. It addresses the challenge of false responses in LLMs.
- Custom Validator Modules: Some frameworks include modules specifically designed to validate source reliability. These modules assess the trustworthiness of online sources used by LLMs.
Ready to go deeper? Recordings from the FP&A series are available to AFP members on AFP Learn. Not yet a member? Join today to get access.
Read More About AI in Finance
Copyright © 2024 Association for Financial Professionals, Inc.
All rights reserved.